The Human Brain Tracks Speech More Closely in Time Than Other Sounds
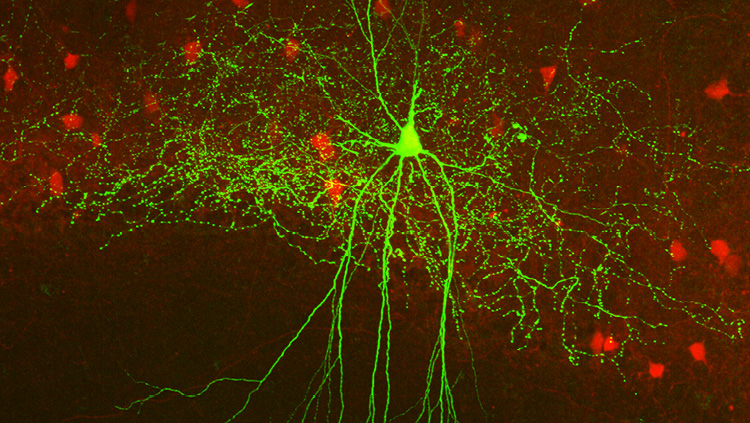
Material below summarizes the article Dynamic Time-Locking Mechanism in the Cortical Representation of Spoken Words, published on June 8, 2020, in eNeuro and authored by Ali Faisal, Anni Nora, Hanna Renvall, Jaeho Seol, Elia Formisano, and Riitta Salmelin.
Highlights
- Computational modeling of cortical responses highlights the importance of accurate temporal tracking of speech in the auditory cortices
- This time-locked encoding mechanism is likely pivotal for transforming the acoustic features into linguistic representations
- No similar relevance of time-locked encoding was observed for nonspeech sounds, including temporally variable human-made sounds such as laughter
![]() |
|
Study Question
How is speech processed differently from other sounds in the brain?
How This Research Advances What We Know
The way that speech processing differs from the processing of other sounds has long been a major open question in human neuroscience. We aimed to answer this by investigating brain representations for natural spoken words (e.g. the word cat) and environmental sounds that refer to the same concepts (e.g. a cat meowing) with the help of machine learning models. Previous studies have shown that most areas that are implicated in processing spoken language are shared with processing other sounds. Machine learning studies using fMRI and intracranial recordings have been able to predict and reconstruct acoustic and phonetic features of a sound based on activation around the primary auditory cortex. However, this is the first study to directly compare decoding of naturally varying speech and nonspeech sounds with computational models that differ in temporal resolution.
Experimental Design
Cortical brain responses were recorded using magnetoencephalography (MEG) while 16 participants listened to spoken words and environmental sounds. The stimuli were spoken words and environmental sounds with corresponding meanings. We utilized the natural acoustic variability of a large variety of sounds (words spoken by different speakers and environmental sounds from many categories) and mapped them to magnetoencephalography (MEG) data using physiologically-inspired machine-learning models. The models differed in how they represented time (time-sensitive versus non-time-sensitive models); the time-sensitive model views the cortical brain responses as closely following, i.e. time-locked to, the time-evolution of the sound features. We decoded acoustic, semantic and (for speech only) phoneme features of the sounds. The models were first trained to learn a mapping between the MEG-responses and the stimulus features. Then, given MEG responses to two test sounds, the mapping was used to differentiate the sound pair in a two-fold cross-validation scheme. Significances were computed by permutation tests.
Results
Comparison of time-resolved and time-averaged acoustic models revealed that speech acoustics were best decoded with the time-sensitive model. The phoneme sequences were also decoded successfully with the time-sensitive model with similar timing as the acoustic features. Thus, the results show that the cortical activation follows the unfolding speech input (each frequency band of the spectrogram) especially closely in time, i.e. is "time-locked" to it. This mechanism seems to be essential in the online mapping from acoustic to phoneme representations for speech encoding. Time-locked encoding was also observed for meaningless new words and for the very beginning of the spoken words, indicating that it is not largely affected by identification of a real word.
As a contrast, time-locking was not highlighted in cortical processing of other sounds that conveyed the same meanings as the spoken words; environmental sounds were best reconstructed with a time-averaged model. Thus, time-averaged analysis seems to be sufficient to reach their meanings. Even responses to human-made non-speech sounds such as laughter did not show improved decoding with the dynamic time-locked mechanism and were better reconstructed using a time-averaged model, suggesting that time-locked encoding is special for sounds identified as speech. Control analyses showed that the differences were not due to acoustic differences, such as higher temporal variability or different modulation content for speech. Semantic features were successfully decoded for both spoken words and environmental sounds, showing that the processing of both types of sounds converged to the same endpoint.
Interpretation
The results suggest that speech is represented cortically in a special manner, where evoked activations follow the sound accurately in time. The present findings deepen the understanding of the computations required for mapping between acoustic and linguistic representations (from sounds to words) and may share a link to cortical tracking of continuous speech that has been observed in other studies. The current findings raise the question of what specific aspects within sounds are crucial for cueing the brain into using this special mode of encoding. Future work could investigate the contribution of different statistical properties within speech acoustics, the possible effect of expertise, and the contributions of top-down effects using real-word sound environments. These machine learning models could also be useful when applied to clinical groups, such as investigating individuals with impaired speech processing.
Visit eNeuro to read the original article and explore other content. Read other summaries of eNeuro and JNeurosci papers in the Neuronline collection SfN Journals: Research Article Summaries.
Dynamic Time-Locking Mechanism in the Cortical Representation of Spoken Words. Ali Faisal, Anni Nora, Hanna Renvall, Jaeho Seol, Elia Formisano, and Riitta Salmelin. eNeuro 8 June 2020, 0475-19.2020; DOI: 10.1523/ENEURO.0475-19.2020