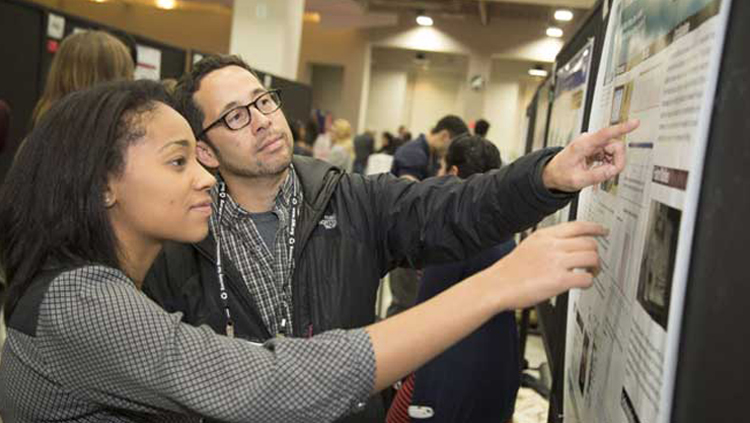
When we open our eyes in the morning, we take for granted that we can consciously see the room in which we went to bed the night before. We likewise take for granted our ability to consciously hear, feel, and know things about the world, and can combine these several kinds of conscious awareness to control actions that realize valued goals in the world.
Many neurobiological experiments have clarified where in our brains conscious states may occur. But how they occur, or perhaps even more consequentially, why evolution may have been driven to discover conscious states in the first place, have remained mysterious until recently.
I was led to scientific answers to these questions because of my fascination with how we learn about the world around us. I discovered my first neural network models as a Freshman at Dartmouth College in 1957, along with the basic laws for how our brains store and learn information. My experience illustrates that no student is too young to do science if they know what new questions to ask.
I have always been astonished by how quickly we learn things, and how long we remember them. For example, after seeing an exciting movie, in which individual scenes flash by quickly, we can go home to tell our family and friends lots of details about it.
I call this problem the stability-plasticity dilemma. How do we learn things quickly but remember them for a long time? Why does a fast-learning rate not force a fast-forgetting rate? Answering this question illustrates the power of neural models.
Why are models needed?
No experimental method can, by itself, show how a brain makes a mind. That is because psychological functions are emergent properties of many neurons interacting across multiple brain regions. Only a neural model can explain how interacting brain mechanisms cause emergent psychological properties.
In 1976, I introduced Adaptive Resonance Theory (ART) to show how adaptive resonances solve the stability-plasticity dilemma. ART was incrementally developed with multiple student collaborators into the most advanced cognitive and neural model that explains how humans learn to attend, recognize, and predict objects and events in a changing world.
Students need assurance that what they are studying is true. ART provides such assurance by providing principled and unifying explanations of hundreds of psychological and neurobiological experiments that other models have not explained.
A deeper form of assurance, and one that can be used to teach all students how ART works, is that I derived ART in 1980 from a thought experiment.
Students may already be familiar from their physics classes with the famous thought experiments that Einstein used to derive Special Relativity Theory and General Relativity Theory.
My own thought experiment asked how ANY learning system can autonomously correct predictive errors in a changing world.
The hypotheses of this thought experiment are facts that are familiar to everyone because they represent ubiquitous environmental pressures on the evolution of our brains. Students do not need to know any science to follow it.
In fact, nowhere during the thought experiment are the words mind or brain mentioned.
ART design principles and mechanisms are thus a universal solution to the stability-plasticity dilemma because it asks how any system can learn quickly without experiencing catastrophic forgetting.
Brain Evolution Achieves Behavioral Success
These models were derived from an analysis of psychological data. Surprisingly, this analysis led to explanations of lots of brain data too.
Why is this? It is because brain evolution needs to achieve behavioral success.
A student who studies only psychology will learn the functions of behavior. A student who studies only neuroscience will learn the mechanisms of the brain.
Without a linkage between them, psychological functions have no mechanistic explanation, and brain mechanisms have no functional meaning.
It is therefore important for students who study brains to consider the psychological properties that brains cause, and how we can use models to study how the properties are caused.
Want to learn more or discuss this topic? Explore the Neuronline Community for more resources.