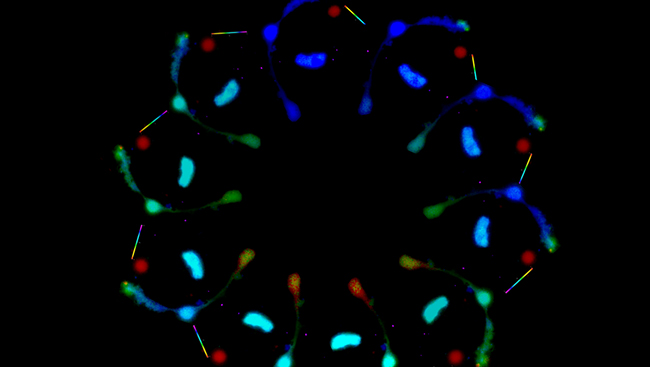
Material below is adapted from the SfN Short Course Scientific Opportunities and Challenges in Single-Cell Analysis, by Steven A. McCarroll, PhD. Short Courses are day-long scientific trainings on emerging neuroscience topics and research techniques held the day before SfN’s annual meeting.
Until recently, analyzing a single cell to determine what genes it expresses and how it functions was beyond reach. But new techniques have emerged within the last 10 years that give researchers this capability, as well as the ability to analyze aspects of many individual cells at once. When combined with analyses of cell shape, connectivity, and activity, probing the genomes and expression profiles of single cells has the potential to yield powerful insights, yet also holds challenges.
Early attempts at single cell analysis hinted at possible applications and piqued scientific interest. As these approaches become more powerful and reproducible, their benefits lie in their widespread use and in the collection of resulting data. For instance, searchable datasets might contain the transcriptomes of many single cells. So called “digital atlases” could make comparisons of gene expression in different cell types quicker, easier, and more accessible than ever before.
Datasets that collect multiple categories of information for individual cells could also generate greater understanding. A recent study combined single-neuron electrophysiological, morphological, and gene expression information into one database with the potential for highlighting new connections between these properties. While promising, one challenge scientists face when attempting to integrate multiple pieces of information about an individual cell is that often one type of analysis destroys the cell, which prevents other types of inquiry.
Another difficulty that scientists face in single cell analysis is in differentiating meaningful biological information from artifacts of the techniques. A connected challenge is determining the best way to quantify the data and to then make accurate comparisons within and between datasets. For example, methods for studying gene expression often include amplification, which can complicate analyses that rely on quantification of the number of reads of a certain sequence detected in an experiment.
One measurement used to determine the validity of transcriptome analysis is the number of genes detected in each cell. This metric is problematic because this count varies widely and depends upon cell type. One way to overcome these issues is to attach a barcode, called a unique molecular indicator, to each complementary DNA before sequencing. Using these indicators allows researchers to quantify individual transcripts and to minimize the effects of amplification. Further development of metrics that take into account technical artifacts and allow for maximum experimental consistency in order to make accurate comparisons will be essential for single cell analysis to be most effective.
The amount of data generated in single cell analyses is often enormous. Computational strategies that work well for the data generated from one individual cell do not always scale up appropriately. Therefore, cultivating interdisciplinary approaches that accurately cope with genome and transcript-level data from thousands of separate cells is necessary. If this challenge can be met, it will provide an opportunity to explore cellular function and gene expression in a way that is unbiased by preconceived notions of what might be found.
How living things function depends upon not only how their cells work, but how their cells work together. Researchers must embrace the opportunity to understand these relationships by both zooming in to look at the genomic, expression, morphological, and connectivity characteristics of single cells and zooming out to compare these attributes across individual cells and cell types.