Looking at Scientific Rigor Opportunities and Challenges
- Featured in:
- Rigor and Reproducibility
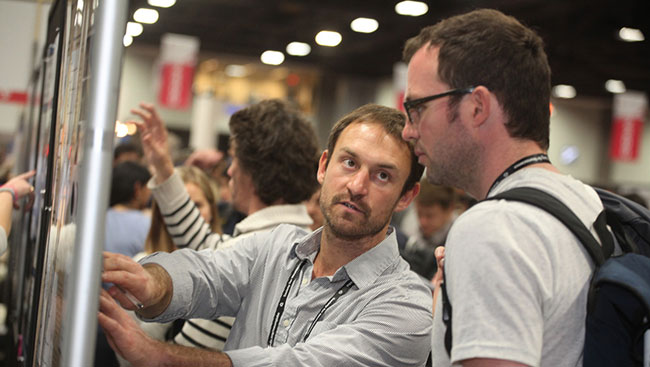
Scientific rigor is about trying to make sure that our experimental design, analytical techniques, and how we interpret, reproduce, and replicate data are strong enough that we can make sure we are on the right path for scientific discovery.
If we don’t have good scientific rigor, it could lead to results that lead us down the wrong path — potentially to dead ends. This outcome is very costly in terms of dollars and time for investigators and funders. We all want to make sure that our science is accurate.
Challenges
Neuroscience is a very noisy enterprise. The experimental procedures and techniques we use, while improving, are not perfect. If work is not done properly and the proper experimental procedures are not conducted, the limitations of our measurements can easily produce Type 1 and Type 2 errors (for example, false positives or negatives) with regard to outcomes.
A secondary result of this phenomena is that the neuroscience literature, too, tends to be a little noisy. While we have lots of new research telling us about the brain and brain function, it doesn't necessarily all accurately portray the underlying brain physiology related to brain function. Good scientific rigor is critical to preventing the noise.
Additionally, with increasing technological advances and downward pressures to get people out of training as soon as possible, it could be argued that there is not enough time for trainees to learn what is necessary scientific rigor.
Another challenge is that it is relatively straightforward to reward investigators for high productivity, such as publishing many papers, because this metric is easy to quantify quickly and it is built into the “psyche” of most review panels. On the other hand, it is much harder to reward investigators for good experimental practices.
Performing research with good scientific rigor often takes more time and limits the total number of publications an investigator may have, and it can lead to negative results, which are often not published or not as flashy to journal editors. And often, but not always, the level of a publication’s scientific rigor is not a top consideration when evaluating if it is good science.
Opportunities
As a field we need to expose young scientists to good scientific practices, help them understand how important it is, and develop a system where we can reward them for that effort.
SfN’s webinar series, Promoting Awareness and Knowledge to Enhance Scientific Rigor, part of NIH’s Training Modules to Enhance Data Reproducibility grant, provides a good foundation for people to expand their knowledge on good scientific practices — from acquiring data to analyzing and interpreting data, as well as becoming familiar with the growing literature on best practices.
In the series’ third webinar, Best Practices in Post-Experimental Data Analysis, which I moderated, I hope that people learn about proper and improper statistical techniques and new computational and experimental procedures that will enhance reproducibility in their data.
It’s also important that people understand other resources that can help them reproduce and make sure that their findings are sound. For example, NIH and other funding bodies and international efforts have massive databases that provide resources for people to do further tests on some of their ideas in ways they wouldn’t otherwise be able to do.