Inferring Causality and Functional Significance of Human Coding Variants
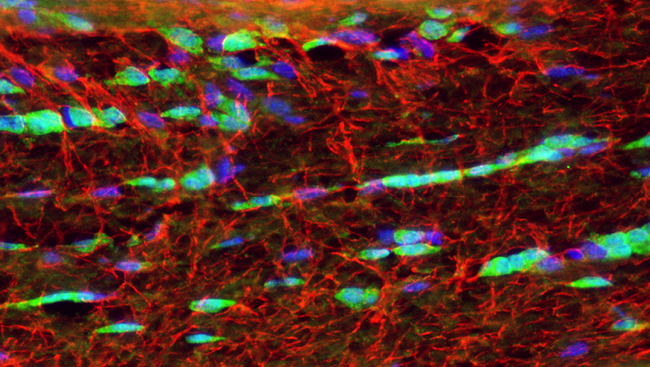
Material below is adapted from the SfN Short Course Inferring Causality and Functional Significance of Human Coding DNA Variants, by Shamil R. Sunyaev, PhD. Short Courses are daylong scientific trainings on emerging neuroscience topics and research techniques held the day before SfN’s annual meeting.
Genetic sequencing technologies allow researchers to characterize the diversity of the human genome as well as the causes of many diseases and disorders. Still, researchers struggle to understand the effects of many identified genetic alterations.
Some changes may be harmless, but others may contribute to phenotypic changes through more complex genetic or biological interactions.
If researchers can identify the exact biological effect a mutation has — how it alters a protein's function, for example — linking disorders and disease to their genetic underpinnings becomes possible.
This course provides an overview of three avenues to help reveal the effect of genetic alterations: Statistical methods can flag potentially important variants, experiments in vitro and in animals can show a gene's function by what happens in its absence, and computational methods can predict how a mutation might affect gene function.
None of these approaches can prove that a genetic alternation causes a particular disruption on their own, but by combining all three researchers can build a strong case for a connection.
For relatively straightforward cases, where a change in a single gene causes a phenotypic change, a statistical association occurs when the variation crops up more often in affected individuals than it does in controls.
However, proving the link is causal requires phenotypic and genetic information from multiple generations.
Data from a large number of unaffected controls can boost the certainty of an association.
Another statistical approach involves comparing the genes of unaffected parents with those of their affected child/children to identify new mutations. Such de novo mutations are prime candidates for further investigation.
Even with a strong statistical association, experimental validation is needed to infer a link.
Experimental approaches include a number of lab-based methods that can show the effect of an alteration at the molecular, cellular, or whole-organism level. But there is always the risk that in vitro and animal experiments will not translate to humans.
It's important to pick the appropriate experimental method.
For example, an alteration that effects how much of a protein is made in the cell can be tested with specific assays, but those same tests may not reveal a problem that arises only when the protein interacts with another protein. Multiple assays may be required.
Computer algorithms allow scientists to use the information they already know about gene and protein networks to predict the biological consequences of specific genetic alterations. While less clear-cut than direct experimental evidence, computer algorithms offer relatively inexpensive way to gain insight.
Available algorithms typically rely on two basic principles.
First, because many proteins developed under evolutionary pressure, comparing human proteins to their analogs in other species can reveal potentially disruptive changes.
Second, harmful mutations frequently disrupt a protein's structure. If an alteration makes a protein's structure unstable, the algorithm can flag it as worth further analysis.
Different algorithms may disagree about the effect of any single alteration because computational thresholds researchers set can greatly affect the outcome. Eventually, more complete biological understanding and data sets will help resolve such disagreements.
Complex traits involving multiple genes as opposed to traits that arise from changes in just one gene are even more challenging. Additionally, many current statistical approaches aren’t adequately powered for very rare variants.
Studies focusing on genes researchers suspect might be involved in a disease based on their function or association with other genes may yield to computational methods that flag candidate genes coupled with experiments to test the result of changes.