Cynthia Chestek on Her Career Bridging Neuroscience and Electrical Engineering
![]() |
|
This resource was featured in the NeuroJobs Career Center. Visit today to search the world’s largest source of neuroscience opportunities.
Cynthia Chestek brings an electrical engineering approach to discover how to allow movement of multiple fingers simultaneously following paralysis or amputation, using a brain-machine interface. In this interview she recounts the moment she knew she’d pursue a career in neuroscience, describes the rewards of that career, and looks to the future of neural interface technology, including neural networks. She also offers advice to women in engineering for a successful graduate education and career.
This article is part of Neuronline’s interview series “Entrepreneurial Women Combining Neuroscience, Engineering, and Tech,” which highlights the career paths and scientific accomplishments of female leaders and role models who are creatively bridging disciplines to improve lives.
How would you describe your work in brain-machine interfaces?
I consider brain-machine interfaces a specific form of neural interfaces. The way I explain it is the nervous system is really a circuit. Tapping into that circuit gives you information about what’s going on with the body, and putting information back into that circuit also lets you treat all kinds of medical conditions.
![]() |
|
My lab records from the nervous system to get control signals for prosthetic hands or for stimulating paralyzed limbs, because that information is still available in nerves or the brain. My work spans the breadth of electrical engineering applied to solving that problem.
For example, we work on algorithms for interpreting neural activity. That includes low-power circuits so we can do this inside of the body, and using electrodes that are smaller than neurons. We want to understand the real neuron-to-behavior translation and implement it with our algorithms to individuate fingers for prosthetic control.
Can you walk us through the highlights of your career path?
I got set on this path during undergrad. I went to college with an idea that I was good at math and studied electrical engineering. A professor suggested that I think about staying in research, and around the middle of undergrad, I found a job in a neuroscience lab working with circuits.
As soon as I spent the summer recording signals from live neurons, I knew I wanted to do that for the rest of my life. The nervous system can be very accurately modeled by circuits, and that became real to me when I was recording these electrical impulses and watching them control muscles. That blew my mind.
As soon as I spent the summer recording signals from live neurons, I knew I wanted to do that for the rest of my life.I ended up going to grad school to study brain-machine interfaces, because if you averaged electrical engineering and neuroscience, that's the topic of equal overlap. I went to Stanford, got my PhD in electrical engineering, and got to record from live neurons every day. I stayed and decided to become a professor because I had the sense that this field was about to explode.
What inspires your work?
I started my scientific life in an Aplysia lab, where you can see the whole system. Starting in that very simple system drove it home that this is a circuit we might be able to understand and use. I still have that initial sense that this is a circuit we can understand, and that there's nothing magical happening. It's direct connections and relatively direct circuit-level control.
This is already having large results for medical conditions. Brain-machine interfaces didn't really exist 20 years ago, and now you can do two-dimensional cursor control almost as well as you can with your real hand.
If you imagine what's going to happen in 20 years from now and, in particular, if you look at initial demonstrations of new technologies we're going to have access to, including cellular-scale electrodes — if we discover nothing else, it's going to be an amazing next decade as these technologies make their way into medical applications.
You can watch your work have an effect, and that makes this a very rewarding field to work in.
Your lab is part of the University of Michigan Biointerfaces Institute, which emphasizes interdisciplinary collaborations, as well as NeuroNex, a part of the BRAIN Initiative advancing neural and cognitive research. How have these collaborations helped you advance your scientific goals?
I sit in a really nice mid-point between the traditional engineering disciplines and medical doctors. I've always been in labs that included a mix of engineers and neuroscientists, and now I have collaborations on both sides, which makes this kind of research a lot of fun.
I've always been in labs that included a mix of engineers and neuroscientists, and now I have collaborations on both sides.In particular, I have very close collaborations with the surgeons I work with on both the brain and peripheral nerves. That sort of frequent contact is critical to making sure what we’re doing will have an impact on people's lives.
NeuroNex and other collaborations are more on the engineering side. None of that will go to humans tomorrow, but it will be applicable to neuroscience studies. For example, we're trying multiple ways of getting dramatically higher channel counts and trying to make very small wireless recording motes.
What challenges have you run into throughout your career? Who or what helped you navigate those?
The most challenging times have been the first semesters of anything new — freshman semester of undergrad, the first semester of my PhD program, my first semester teaching as a professor. It can feel like the difficulty level just doubled on you, and that is the point when everyone questions whether they should be there. But you have to overcome that challenge to succeed at the next one. There has to be a certain faith that there's light at the end of the tunnel, which I had.
I can also point to individual people who helped me keep going through those periods. I had excellent mentors, classmates, and lab mates, at every stage of my career. I was always good at finding people who were interested in being a mentor. Actually, it was a priority for me. The people who are the most useful to talk to are often those who are one year ahead of you or in your same class.
It’s important to integrate with what’s happening around you socially. Get to know people and ask for help when you need it. That gives you the social support that can get you through the challenging times.
What has been your experience as a woman in a traditionally male-dominated field?
![]() |
|
The challenging periods are always full of deep self-doubt, and knowing you’re the only woman in the room can add an extra level of stress, which is likely invisible to male colleagues. This contributes to impostor syndrome and stereotype threat, the feeling that you're representing all women. If you're looking at why there are fewer women in the field, you have to look at what they’re experiencing during those periods of peak stress.
I understand the social discomfort of being in male-dominated classrooms and spaces. That said, I’ve had great professional relationships with my mentors and cohorts, and they’ve been almost universally men. If you're comfortable with that, and I was, you can find people to encourage you when you really need it in order to get through the hard parts.
What’s your biggest piece of advice for women considering a similar career?
I can also point to individual people who helped me keep going.
If I could say one thing to women starting in engineering programs, it's that guys don't tell you when they're having trouble. A woman might have the same or much better grades than other people who are struggling with the same material and still feel like she's the only one struggling, based on cultural differences between how men and women talk about having problems. It's a small cultural difference that has a big impact.
Use numbers to see whether or not you're doing well, and do homework with other people so you know that everybody's having the same difficulties that you are.
Where do you see brain-machine interfaces going?
In a few decades, the present day may be seen as the age when all of this started working. And that's, I think, due to this sort of long-sighted vision that if you invest in the technology to talk to neurons, that has wide-range implications for what comes next.
There have been no demonstrations of brain-machine interfaces using state-of-the-art deep learning, partially because it's computationally complex and partially because these algorithms overfit. By overfit, I mean that it will crash in the face of any slight change, and whenever you're trying to do something in real time, there will be slight differences between your training data and what's happening.
But there is universal agreement that neural networks and other nonlinear approaches should enable us to do things that we haven't done before. I think we haven't yet seen the full effect that deep learning and neural networks are going to have on brain-machine interfaces.
Speaker
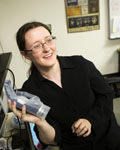