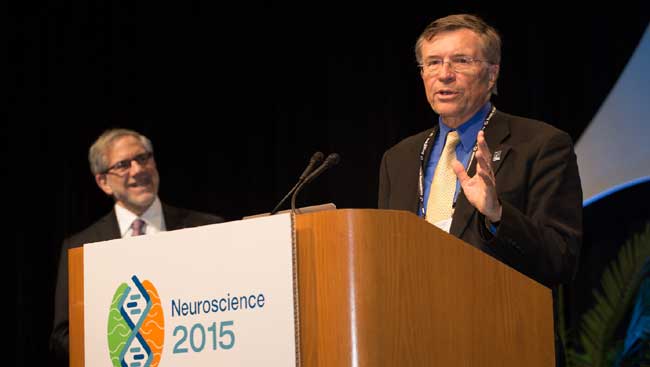
Terrence Sejnowski of the Salk Institute for Biological Studies is a pioneer of computational and theoretical neuroscience. He developed artificial neural network models and learning models for birdsong and neuroeconomics. He also helped develop the algorithm for independent component analysis. For these and other accomplishments, Sejnowski received the Swartz Prize for Theoretical and Computational Neuroscience in 2015. Here he reflects on his career and where the field is headed.
What discoveries made in your lab have most excited you and why?
Recently my lab has focused on high-resolution EM reconstructions, which we call nanoconectomics, and particle models of molecular interactions at synapses. We recently showed that cortical synapses have more than 26 distinguishable sizes corresponding to five bits of information storage, an order of magnitude greater than previous estimates. Why is the precision so high when on a given input, synaptic release is so unreliable? In the process of studying dysfunction in cortical circuits, we collaborated with Joe Ecker to sequence the pattern of DNA methylation in neurons and discovered a unique form of methylation in neurons during brain development. This is an exciting new direction to look for the cause of developmental diseases in the brain.
What is most interesting to you about where the field of computational neuroscience is headed?
There is a tremendous opportunity in analyzing and modeling data from human brains. I have been collaborating with Syd Cash and Eric Halgren to analyze cortical recordings from human epilepsy patients. My lab recently discovered that cortical sleep spindles are not synchronous across the cortex, but are global, circular traveling waves. There is evidence that these global patterns of spindle activity contribute to memory consolidation.
What do you think it will take to accelerate progress?
Richard Feynman once said that you have not understood something until you can build it. In the 1980s, I worked on learning algorithms for multi-layer neural network models with Geoff Hinton that could explain the diversity of response properties that are found in populations of neurons, which we called distributed representations. The tremendous increase in computer power since then has made it possible to build deep networks with many layers that are approaching human levels of performance in speech recognition and object recognition in images. In the 1990s, we modeled the dopamine system using temporal difference learning, which led to a deeper understanding of reward systems in the basal ganglia. The power of reinforcement learning when coupled with deep learning was demonstrated recently when AlphaGo beat Lee Sedol, a world champion at Go. These network architectures are caricatures of brains based on 20th century neuroscience, but as the BRAIN Initiative accelerates, we should be able to build new architectures with even more impressive capabilities.
You once told Cognitive Science Online, “At the highest levels of achievement in science one often finds the highest levels of persistence.” How have you stayed resilient and focused as your research trajectory unfolds?
In my experience, the determining factor in the success of most projects is the degree of motivation you have to get to the bottom of the problem. If you are passionately involved, you are much more likely to reach a solution. But the most important advances have been surprises along the way, something that you cannot plan or predict. I have been fortunate to have great students and colleagues who have been highly motivated to make progress working together.
In growing the field of computational neuroscience, you have mentored and trained countless scientists. What skills and qualities most benefit trainees in this area?
The most important decisions are made at the outset of the research, picking a question that is important but might yield to the right approach. The skills you need to answer the question depend on the approach, whether it be experiment, modeling, or theory. Francis Crick once advised me that I should not become too fond of a model, because it was not an end in itself but a means to design a killer experiment that gives the game away. Models can provide a better intuitive understanding of a complex system like the brain, but only experiments can provide the answers.
Speaker
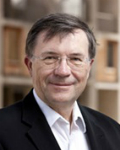