On the Ethics of Machine Learning Applications in Clinical Neuroscience
- Source: The Neuroethics Blog
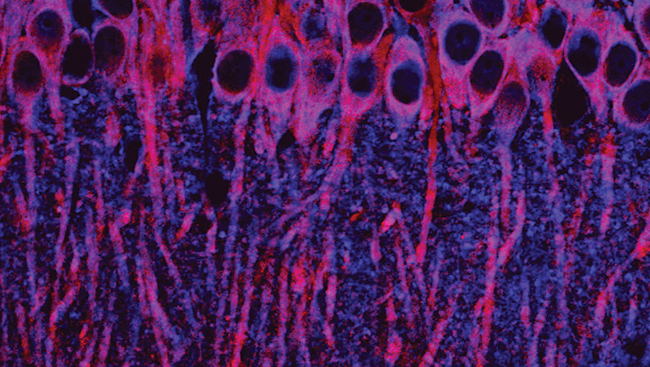
Machine learning refers to software that can learn from experience and is thus particularly good at extracting knowledge from data and for generating predictions.
Recently, one powerful variant, deep learning, has become the staple of recent progress and hype in applied machine learning. Deep learning uses biologically inspired artificial neural networks with many processing stages. These deep networks, together with the ever-growing computing power and larger datasets for learning, now deliver groundbreaking performances.
For example, Google’s AlphaGo program, which comprehensively beat a Go champion in January 2016, uses deep learning algorithms for reinforcement learning (analyzing 30 million Go moves and playing against itself).
Despite these spectacular and media-friendly successes, the interaction between humans and algorithms may also go badly awry.
The software engineers who designed Tay, the chatbot based on machine learning, surely had high hopes that it may hold its own on Twitter’s unforgiving world of high-density human microblogging. However, these hopes turned to dust when seemingly coordinated interactions between Twitter users and Tay turned the ideologically blank slate of a program into a foul display of racist and sexist tweets.
These examples reflect diverse efforts to create more and more “use-cases” for machine learning, such as predictive policing (using machine learning to proactively identify potential offenders), earthquake prediction, self-driving vehicles, autonomous weapons systems, and the composition of Beatles-like songs or lyrics.
Here, I focus on some aspects of machine learning applications in clinical neuroscience that, in my opinion, warrant particular scrutiny.
Machine Learning Applications in Clinical Neuroscience
In recent years, leveraging computational methods for the modeling of disorders has become a fruitful strategy for research in neurology and psychiatry.
For example, in clinical neuroimaging, machine learning algorithms have been shown to detect morphological brain changes typical of Alzheimer’s dementia, identify brain tumor types and grades, predict language outcome after stroke, and distinguish typical from atypical Parkinson’s syndromes.
In psychiatric research, examples for applying machine learning are the prediction of outcomes in psychosis and the persistence and severity of depressive symptoms.
Generally, most current applications follow one of these rationales: distinguish between healthy and pathological tissue in images, to distinguish between different variants of conditions, or to make predictions on the outcome of particular conditions.
While these are potentially helpful tools for assisting doctors in clinical decision-making, they are not yet routinely used in clinics. It is safe to predict, however, that machine learning based programs for automated image processing, diagnosis, and outcome prediction will play a significant role in the near future.
Some of the Ethical Challenges
One area in which intelligent systems may create ethical challenges is their impact on autonomy and accountability of clinical decision-making. As long as machine learning software for computer-aided diagnosis merely assist radiologists, and the clinician keeps the authority over clinical decision-making, it would seem that there is no profound conflict between autonomy and accountability.
If, on the other hand, decision-making was to be relegated to the intelligent system — to any degree whatsoever — we may face the problem of an accountability gap. After all, who or what would need to be held accountable in the case of a grave system error resulting in misdiagnosis: the software engineer, the company, or the regulatory body that allowed the software to enter the clinic?
Another problem may arise from the potential for malicious exploitation of an adaptive, initially “blank” machine learning algorithm, as in the case of Tay, the chatbot. A machine learning software in its initial, untrained state would perhaps be vulnerable for exploitation by interacting users with malicious intents.
Nevertheless, it still requires some leap of the imagination to go from collectively trolling a chatbot to become racist or sexist to scenarios referred to as neurohacking, in which hackers viciously exploit computational weaknesses of neurotechnological devices for improper purposes.
Despite this potential for misuse, the adaptiveness of modern machine learning software may, with appropriate political oversight and regulation, work in favor of developing programs that are capable of ethically sound decision-making.
While intelligent systems based on machine learning software perform increasingly more complex tasks, designing a moral machine (also see previous discussion) — a computer program with a conscience —remains elusive. A rigid set of algorithms will most likely perform poorly in the face of uncertainty and in ethically ambiguous or conflicting scenarios, and they will not improve behavior through experiences.
From an optimistic point of view, the “innate” learning capabilities of machine learning may enable software to develop ethically responsible behavior if given appropriate data sets for learning. For example, having responsible and professionally trained humans interact with and train intelligent systems — digital parenting — may enhance the moral conduct of machine learning software and immunize it against misuse.
While the limited scope here precludes an in-depth reconstruction of this debate, I encourage you to ponder how the extent of and relationship among autonomy, intentionality, and accountability, when exhibited by an intelligent system, may influence our inclination to consider it a moral agent.
Meanwhile, one interesting ancillary benefit that arises from this increasing interest in teaching ethics to machines is that we study the principles of human moral reasoning and decision-making much more intensely.
Suggestions for Political Regulation and Oversight of Machine Learning Software
To prevent maladaptive system behavior and malicious interference, close regulatory legislation and oversight which appreciates the complexities of machine learning applications in medical neuroscience is necessary.
In analogy to ethical codes for the development of robotic systems — responsible robotics — I would emphasize the need for such an ethical framework to include non-embodied software — “responsible algorithmics.”
From the policy-making perspective, the extent of regulatory involvement in developing intelligent systems for medical applications should be proportionate to the degree of autonomous system behavior and potential harm caused by these systems.
We may also consider whether the regulatory review process for novel medical applications based on machine learning should include a specialized commission with experts in clinical medicine, data and computer science, engineering, and medical ethics.
Instead of merely remaining playful children of the Internet age, we may eventually grow up to become “digital parents,” teaching intelligent systems to behave responsibly and ethically — just as we would with our actual children.
Acknowledgments
I thank Julia Turan (science communicator, London, @JuliaTuran) and the editors of The Neuroethics Blog for valuable discussions of the text and editing. I also thank Robin Schirrmeister (department of computer science, University of Freiburg) for clarifications and discussions on machine learning. Remaining factual and conceptual shortcomings are thus entirely my own.
This post is reprinted with permission and originally appeared on The Neuroethics Blog, hosted by the Center for Ethics, Neuroethics Program at Emory University.