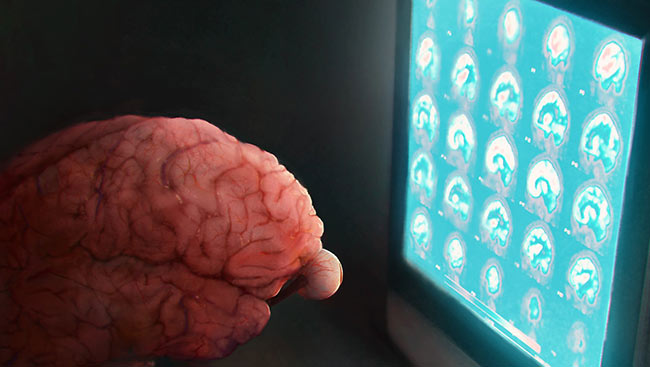
The following case study is adapted from a Theme J abstract presented at Neuroscience 2016. Theme J abstracts cover topics related to history, teaching, public awareness, and societal impacts in neuroscience, allowing departments and organizations to showcase the work they have done in these areas.
Big data offer exciting opportunities to derive insights and make synergistic connections across many levels of inquiry. It has the potential to inform the research community, biomedical practitioners, educators, and even allow avenues for citizen science. However, unlocking the true potential of big data relies on making it usable, which depends on the skill and expertise of scientists collecting, sharing, and analyzing the data.
Since there are only a few educational programs that focus on big data training, we organized the iNeuro Workshop (with the help of NSF funding) to discuss ways to effectively educate neuroscientists to handle big data.
The iNeuro Workshop brought together neuroscientists, library and information scientists, computer scientists, bioinformatics scientists, administrators, and educators to discuss potential educational programs and to chart a roadmap to create a workforce with the skills necessary to work with big data now and in the future.
Our discussion focused on three questions: What are we going to teach? How are we going to teach it? How are we going to evaluate our curricula?
Here’s what we found.
What are we going to teach? Computational and collaboration skills.
All of the biological sciences, including neuroscience, are becoming more quantitative, and to keep up with the field, students will need to apply quantitative methods, including computational modeling, simulation, and data visualization.
Additionally, as we investigate increasingly complex questions, neuroscientists will need to work in teams, which requires the ability to communicate and collaborate across disciplines.
Looking to stakeholders for guidance, we identified the following skills that should be taught:
-
Computational and modeling skills, including data visualization
-
How to curate, translate, steward, and hack the data
- Library and informational sciences
- Teamwork and team building
How are we going to teach it? Exploring genuine research questions with team-based tasks.
Studies show that student performance is enhanced with active learning. Successful STEM programs frequently engage students in genuine research (and this seems particularly important to encourage diversity in STEM programs).
Teaching should engage students as active participants in inquiry driven courses and integrate research experiences as essential components of the curriculum.
I find that when I teach lab using genuine research questions, students are a lot more engaged.
Your course should include:
-
Active, multidisciplinary, and team-based teaching and learning
-
Genuine and compelling challenges in neuroinformatics
-
Real data sets in instruction
How are we going to evaluate the instruction and curriculum? Multiple forms of assessment.
Assessments based on student outcomes can reveal the efficacy of the programs and steer improvement. Evaluation should integrate multiple forms of assessment to track student learning, provide students with multiple forms of feedback, and measure outcomes to improve learning environments.
Design multiple forms of assessment with the desired outcomes in mind and make sure your assessment contains:
- Clear and measurable outcomes stated at the outset
- Measures of how well students develop the concepts and skills deemed most important and foundational
- Metrics such as the number of students applying to the program and completing the degree.
- Outcomes such as research catalyzed, methodologies devised or improved, data sets created or analyzed, presentations given, publications, and placements of graduates
The need to train and educate a workforce to manage, catalog, curate, and effectively use big data is clear. If we update our educational programs to teach these skills, we will achieve the tremendous promise and gain the full value of big data.
*The iNeuro project was supported by NSF grant #1441416.
*Photo by Angela He.